SmolLM2 represents a significant leap forward in artificial intelligence capabilities, showcasing notable enhancements over its predecessor, particularly in areas such as instruction following, reasoning, and mathematics.
Hugging Face’s documentation highlights that the model’s largest variant was trained on an impressive dataset of 11 trillion tokens, utilizing a blend of diverse sources including FineWeb-Edu and specialized mathematics and coding datasets. This extensive training enables SmolLM2 to excel in a variety of performance metrics, allowing it to tackle complex tasks with greater proficiency.
In the context of an industry increasingly focused on large language models (LLMs), the challenges associated with their computational demands have become more pronounced. Leading companies like OpenAI and Anthropic continue to push the envelope with massive model sizes, yet this trend has sparked a growing need for efficient, lightweight AI solutions capable of operating on local devices.
SmolLM2 offers a fresh perspective, delivering powerful AI functionalities that are accessible to a wider audience, including small businesses and independent developers who may find the costs of cloud computing prohibitive.
The reliance on large models often creates hurdles for smaller organizations looking to adopt advanced AI technologies. The operational requirements for these extensive models can result in slow response times, heightened data privacy concerns, and substantial costs—issues that are particularly burdensome for smaller enterprises.
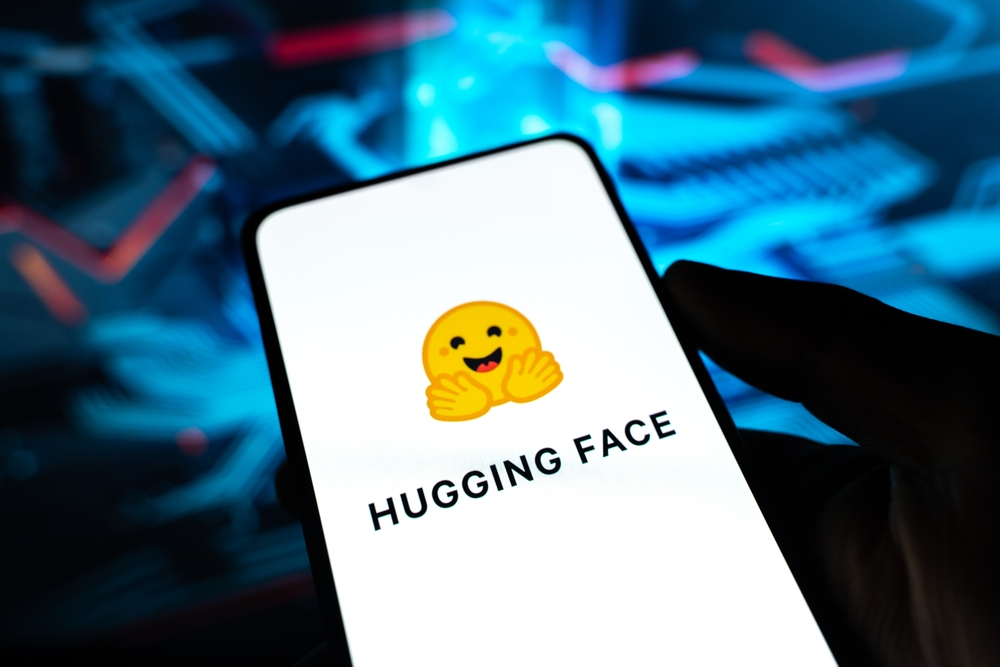
SmolLM2 Revolutionizes AI with Enhanced Instruction Following and Local Device Capabilities
SmolLM2’s design seeks to overcome these barriers by enabling robust AI capabilities to run directly on personal devices, which could democratize access to advanced AI tools beyond just the major tech giants with vast data centers.
Comparative analyses highlight SmolLM2’s impressive efficiency, as it achieves competitive performance with fewer parameters than its larger counterparts like Llama3.2 and Gemma. For instance, the 1.7 billion parameter version of SmolLM2 earned a score of 6.13 on the MT-Bench evaluation, demonstrating strong chat capabilities.
Additionally, its 48.2 score on the GSM8K benchmark reflects its effectiveness in mathematical reasoning tasks, challenging the common assumption that larger models are always superior. This finding emphasizes the significance of thoughtful model architecture and careful data selection over mere size.
While SmolLM2 introduces promising advancements, it also comes with limitations, such as primarily supporting English language content and occasional inaccuracies in factual or logical consistency. Nonetheless, the launch of SmolLM2 indicates a crucial shift in AI development, suggesting that smaller, efficient models can provide substantial performance benefits.
By allowing sophisticated language models to run on local devices, SmolLM2 not only broadens access to AI but also mitigates concerns regarding the environmental impact of large-scale AI implementations. The model is now available through Hugging Face’s model hub, with various size variants designed for both basic and instruction-tuned applications.
