Scaling AI projects from successful pilots to enterprise-wide deployments presents significant challenges, particularly in balancing costs against return on investment (ROI). Kareem Yusuf, IBM’s senior VP of product management and growth, points out that the expenses tied to AI—especially costs like token usage when querying inference engines—can quickly undermine the financial benefits of AI projects.
Yusuf stresses that, although pilot projects might yield promising results, organizations must evaluate the ROI in the context of ongoing operational costs if they intend to implement AI at scale.
Yusuf sees decision support as a prime use case for enterprise AI, which can help businesses analyze large datasets and extract actionable insights. This application of AI enables more efficient decision-making by processing data volumes that humans cannot analyze as quickly or effectively.
However, enterprise users are particularly concerned about maintaining data integrity, security, and accuracy. Yusuf emphasizes that AI must be trustworthy, capable of safeguarding data, and free from “hallucinations” or errors, which can erode user trust in AI systems. Therefore, the quality of data and security assurances are critical factors for companies aiming to integrate AI solutions.
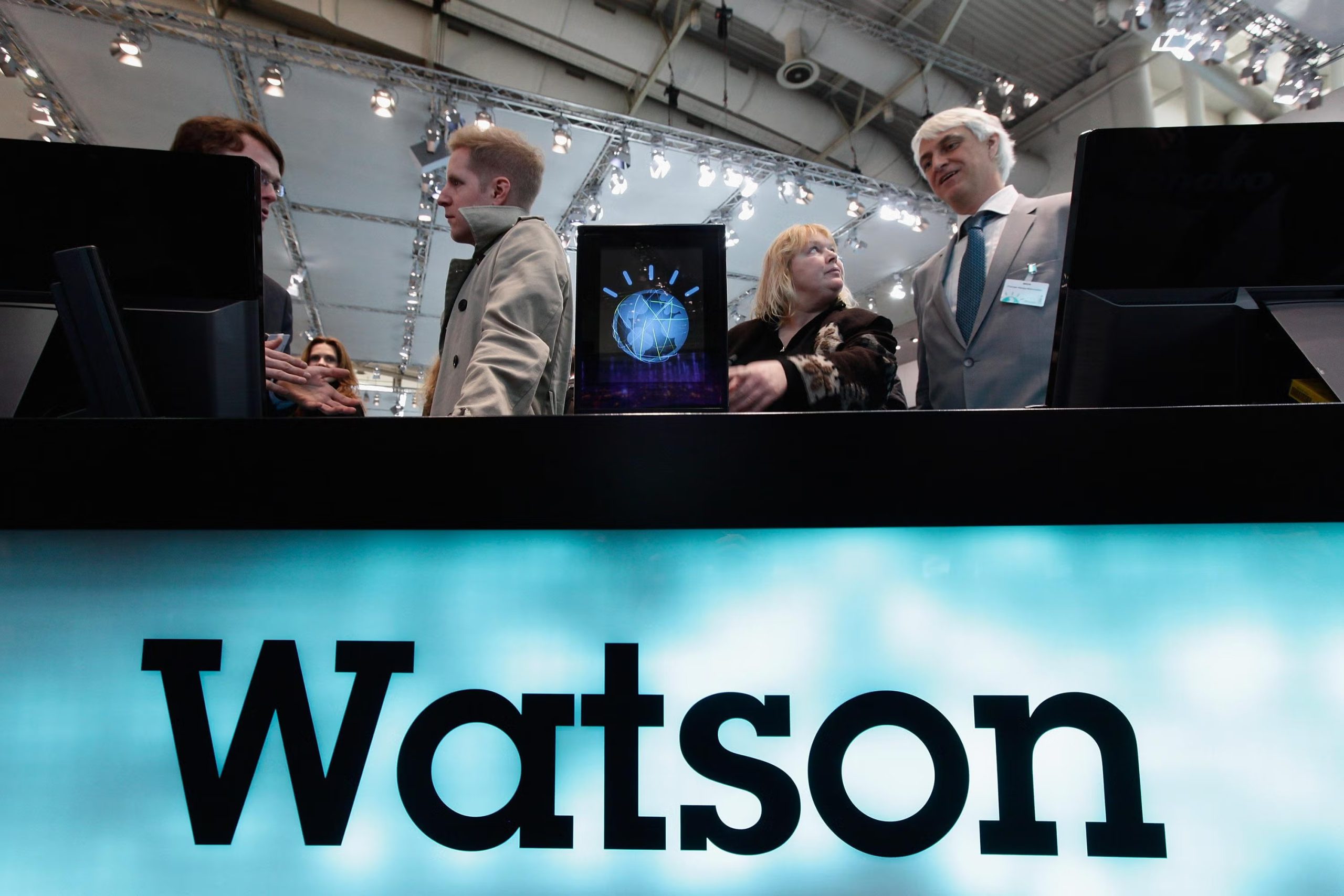
Scaling AI Projects from Pilot Success to Enterprise Deployment Poses Challenges in Cost and ROI Management
Integrating AI into enterprise IT systems adds another layer of complexity, as AI must fit seamlessly within existing infrastructures. Companies face the challenge of ensuring that their AI systems are robust enough to operate at scale while delivering high-quality, dependable outputs.
Yusuf advises leaders to consider these integration needs when planning for AI deployment, as successful implementation depends on the system’s ability to work cohesively with the enterprise’s overall IT architecture. Without careful integration, scaling up AI projects may result in unexpected technical and financial setbacks.
Moreover, organizations must carefully calculate the ROI of AI projects, with Yusuf highlighting that scalability is directly tied to achieving value. In pilot projects, AI often operates in simplified setups that minimize costs, but these expenses can multiply when deployed across an entire enterprise due to usage-based fees and data handling requirements.
Yusuf encourages leaders to ask if a pilot project’s gains will translate to a full-scale deployment, given the real-world costs and challenges of scaling. Achieving reliable, quality results from scaled AI projects is essential for businesses hoping to improve productivity and efficiency.
IBM is positioning itself to meet enterprise AI needs through innovations like InstructLab and Granite 3.0, designed to enhance data protection, integration, and cost-effectiveness. InstructLab offers tools for organizations to integrate customizations into large language models without requiring complete model retraining, saving resources and time.
Additionally, IBM’s Granite 3.0 provides a lower-cost AI solution tailored to business use, significantly reducing operational costs compared to general-purpose models. This focus on smaller, high-performance models reflects IBM’s approach to making AI more accessible and efficient for enterprises looking to capitalize on AI’s potential without overwhelming financial commitments.
