Amazon’s AWS AI team has introduced RAGChecker, a new tool aimed at solving a persistent challenge in AI: ensuring accurate retrieval and integration of external knowledge in AI-generated responses. This tool is particularly important for AI systems that rely on real-time information, such as chatbots and AI assistants, which need to provide up-to-date and contextually accurate information beyond their initial training data.
RAGChecker offers a sophisticated evaluation framework for Retrieval-Augmented Generation (RAG) systems, which combine large language models with external databases. The framework breaks down AI-generated responses into individual claims and assesses their accuracy and relevance based on the retrieved information, offering a more detailed and precise evaluation compared to traditional methods.
This approach helps to diagnose errors in both the retrieval and generation stages, providing a clearer understanding of how AI systems process and utilize external information.
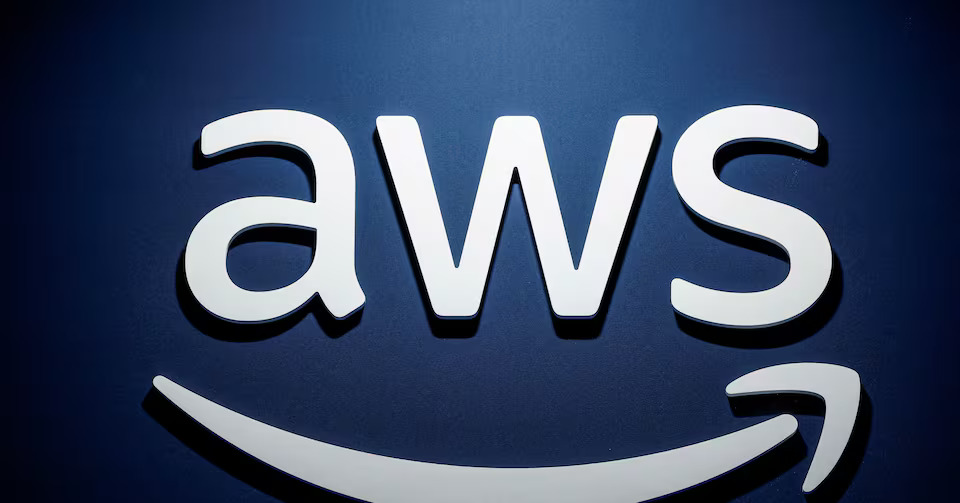
Amazon’s AWS AI Team Introduces RAGChecker for Enhancing Accuracy in AI-Generated Responses
The tool is currently being used internally by Amazon’s researchers, with no public release yet announced. However, if RAGChecker becomes available, it could be integrated into AWS services or offered as an open-source tool for researchers and developers. This framework has the potential to significantly enhance the evaluation and refinement of AI systems, especially in industries where accurate and up-to-date information is critical.
RAGChecker addresses both retrieval errors, where the system fails to find the most relevant information, and generation errors, where the system inaccurately uses the retrieved data. The framework was tested across multiple RAG systems in critical domains such as medicine, finance, and law, revealing trade-offs between accuracy and relevance.
For instance, systems that are strong at retrieving information often pull in irrelevant data that confuses the generation process, highlighting the need for further refinement.
As AI continues to be adopted for high-stakes applications, tools like RAGChecker will become vital in ensuring reliability and accuracy.
By providing a more detailed evaluation of AI systems’ performance, RAGChecker offers valuable insights for improving AI-generated content, especially in industries where errors can have serious consequences. The AWS AI team believes that the metrics provided by RAGChecker will help guide future advancements in AI systems across various sectors.
